Published! The Njoo, McMahan and Akl Labs Publish in ScienceDirect
- ASDRP Admin
- Oct 30, 2024
- 2 min read
Machine Learning Could Drastically Cut Down Time and Cost Needed to Evaluate Fluorine-Based Pharmaceuticals
- - - - - - - - - - - - - - - - - - - - - -
A New Paper from ASDRP's Njoo Research Group, McMahan Research Group and the Akl Research Group shows development in research regarding machine learning. The Njoo anbd McMahan Labs Publish in ScienceDirect.
In a research study recently published to the scientific journal ScienceDirect the Njoo Research group, headed by Edward Njoo, Ph.D. (Stanford University) ASDRP's Director of Research and Chair of the Department of Chemistry and Biochemistry, Dr. Larry McMahan, Chair of the Computer Science Department and ASDRP Advisor and Dr. Marx Akl, ASDRP Advisor, tested different machine learning software and their relative accuracy in predicting the values of 19F (fluorine) based compounds and found there were ways to succeed in achieving impressively accurate numbers comparable to established formulas using softwares that lower the computational cost from hundreds of seconds to milliseconds. Read further for the abstract written by the research group themselves.
The Abstract The Njoo, McMahan and Akl Labs Publish in ScienceDirect.
The introduction of fluorine in compounds plays a crucial role in drug development as it greatly influences their final pharmacokinetic and dynamic properties. Due to the prevalence of fluorine in FDA-approved drugs in recent years, identifying the mechanisms driving their chemical transformations has become crucial in the drug discovery landscape. 19F NMR spectroscopy is a powerful analytical technique that allows for the examination of fluorine-containing compounds, offering valuable information about their structure, dynamics, and reactivity. NMR spectra can be interpreted through the leveraging of Density Functional Theory (DFT). However, the screening of compounds and discovery of feasible drug candidates is limited due to its computational cost. Here, we present a machine learning approach to accelerate the prediction of DFT-calculated 19F NMR chemical shifts. The fluorine atoms’ features in the models were derived from their local three-dimensional environments, representing their neighboring atoms within a radius of n Å away from the given fluorine atom in the compound. A comparative analysis of thirteen regression models was conducted using features extracted from 501 fluorinated compounds in our laboratory’s chemical inventory. Among the models, Gradient Boosting Regression (GBR) exhibited the highest performance, achieving a mean absolute error of 3.31 ppm with a local environment radius of 3 Å. This demonstrates a comparable accuracy to DFT calculations while reducing computational time from several hundred seconds to milliseconds. 3 Å was also found to be the most optimal radius across all models when encoding features for local atomic environments.
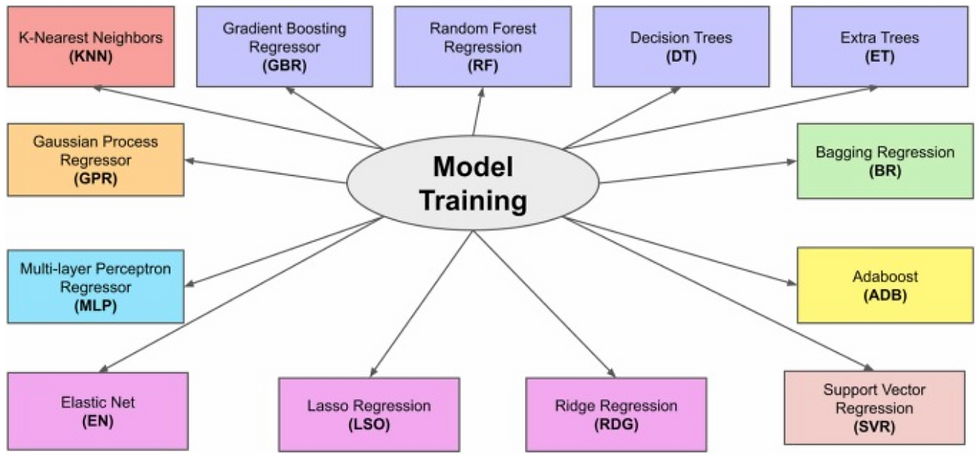
The Team Behind The Study
Sophia Li, BASIS Independent Silicon Valley '25; Emma Wang, Sequoia High School '26; Leia Pei, Hillsdale High School '25; Sourodeep Deb, Mission San Jose High School '26; Prashanth Prabhala, Irvington High School '26; Sai Hruday Reddy Nara, Leigh High School '26; Raina Panda, Washington High School '26; Shiven Eltepu, California High School '26; Marx Akl, ASDRP Advisor; Larry McMahan, ASDRP Advisor; and Edward Njoo, ASDRP Advisor
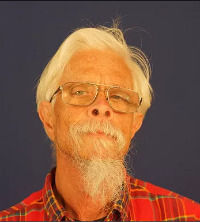
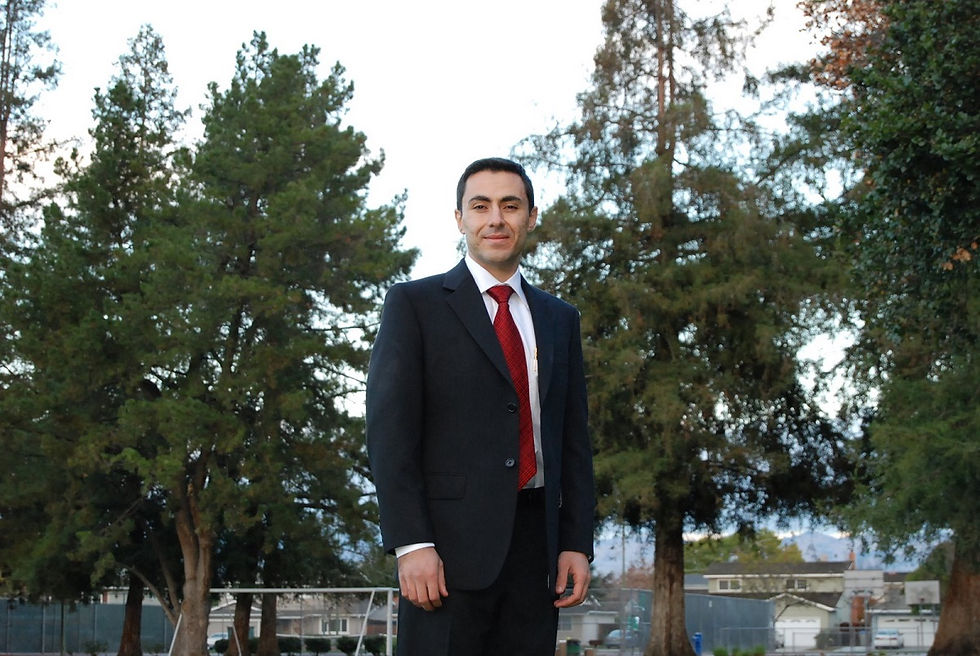
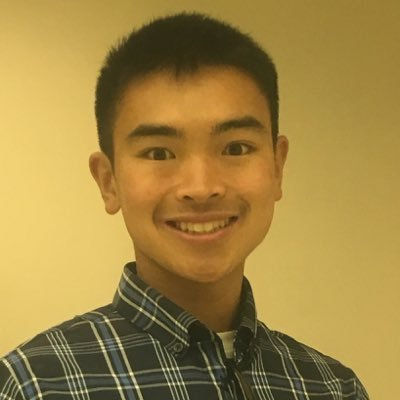
Read More
You can view the full journal publication here.
Comments