Today June 30, we will have four amazing presentations during Colloquia. Visit the Colloquia Site
Department of Computer Science & Engineering
Hybrid Quantum-Classical Graph Generative Models To Target Alzheimer's Disease
Contemporary drug discovery and development processes require billions of dollars and lengthy amounts of time, which is why researchers are utilizing computational chemistry methods like machine learning to speed up molecular synthesis pathways. Alzheimer's disease presents a specific challenge, as treatment requires molecules that can penetrate the blood-brain barrier (BBB). There are currently no effective cures for Alzheimer's disease. Our group is currently working on multiple models utilizing various machine learning techniques to generate chemically stable, novel, and druglike molecules that are of the right size and fit the molecular descriptors to penetrate the BBB. In previous work, our group developed QNetGAN v2, a model that utilizes quantum computing and generative adversarial networks (GANs) to generate chemically feasible molecules for general drug discovery with an 89.23% success rate, generating 116 out of 130 structurally valid molecules. In addition to building off the GAN structure used in our original model to target BBB permeability, our group is working on several other novel models, including message passing neural networks (QMPNN) and several variational auto-encoder (VAE) based methods.
RESEARCHERS: Linda Chang, Homestead High School '25, Nitya Pisolkar, Archbishop Mitty High School '27, Sahil Vijay, Leigh High School '26, Narasimhan Prasana, Mission San Jose High School '25
ADVISOR: McMahan, Quantum Computing & Computer Science
KEYWORDS: Machine Learning | Quantum Computing | Drug Discovery | Computational Chemistry | Alzheimer's Disease
Department of Computer Science & Engineering
Theoretical Study of Quantum Dots in Noise-Resistant Quantum Key Distribution Systems
Quantum key distribution (QKD) systems hold the potential for secure communication by leveraging the laws of quantum mechanics. However, challenges arising from noise and environmental interactions can compromise the security and efficiency of QKD systems. This research aims to explore the theoretical aspects of utilizing quantum dots within noise-resistant QKD systems. By leveraging advanced simulation techniques on a high-performance computing cluster, we investigate the impact of quantum dots on enhancing the security and reliability of QKD systems.
RESEARCHERS: Prahlad Saravanapriyan, Washington High School '25, Praneel Samall, Academy of the Canyons '25, Aditya Das, American High School '26, Samuel L., Saint Francis High School '26, Ezana Makonnen, Archbishop Mitty High School '26, Sophia Ren, The Madeira School '26, Dhanya Ganesh, Basis Independent Fremont '27, Elaine Huang, The Harker School '28
ADVISOR: McMahan, Quantum Computing & Computer Science
KEYWORDS: Quantum Dots | Quantum Key Distribution
Department of Computer Science & Engineering
Diagnosing different types of skin cancer using Machine Learning (CNNs)
Skin cancer poses a significant health challenge, with early diagnosis crucial for effective treatment. Inspection by dermatologists remains the standard for diagnosis, but it can be subjective and prone to error. This project explores the potential of convolutional neural networks (CNNs) as a powerful tool for skin lesion classification, aiming to improve accuracy and accessibility of diagnosis and ultimately contribute to better patient outcomes. Leveraging the data of over 10,000 images of pigmented skin lesions categorized into seven diagnostic classes (including benign and malignant tumors), we will use a deep learning model based on CNNs and Text based models. This project showcases the power of deep learning and multimodal models in revolutionizing skin cancer diagnosis.
RESEARCHERS: Anav Bordia, Basis Independent Silicon Valley '25, Ayaan Chawla, San Ramon Valley High School '25
ADVISOR: McMahan, Quantum Computing & Computer Science
KEYWORDS: Machine Learning | Skin Cancer | Multimodal
Department of Chemistry, Biochemistry & Physics
Discovery, Synthesis, and Optimization of 5-phenylisoxazole Based Covalent Inhibitors Targeting G12C Mutant KRAS for the Treatment of Cancer and Optimized Preparation of Nucleozin and analogs for Influenza inhibition: A unified strategy for challenging amide coupling reactions in medicinal chemistry
RESEARCHERS: Natalie Brahan, Irvington High School ‘25
ADVISOR: Njoo Lab Synthesis | Physical Organic Chemistry | Catalysis | Chemical Biology | Spectroscopy | Medicinal Chemistry
KEYWORDS: More info to come
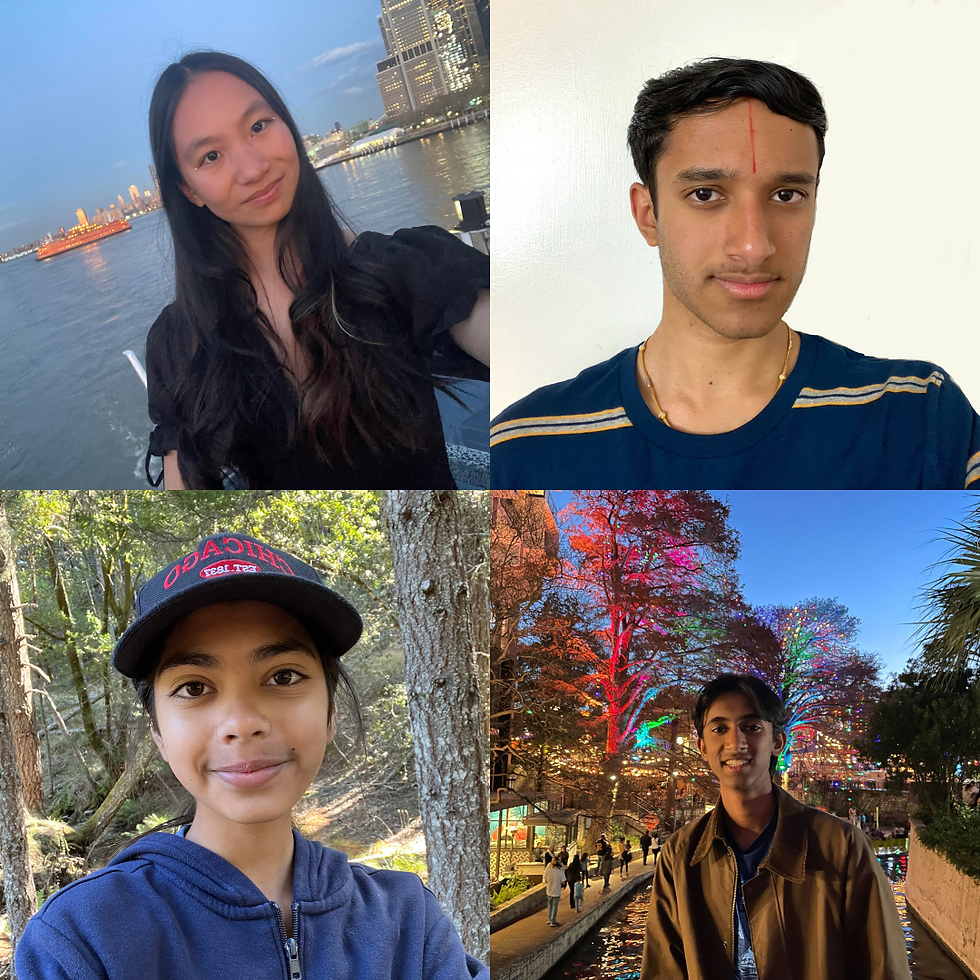
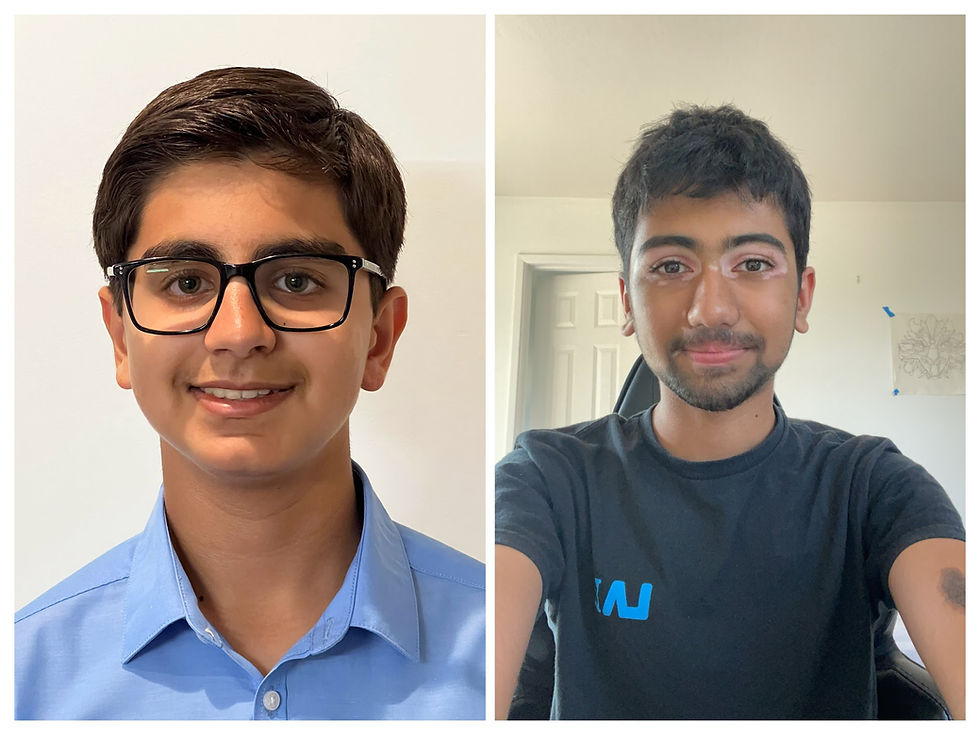
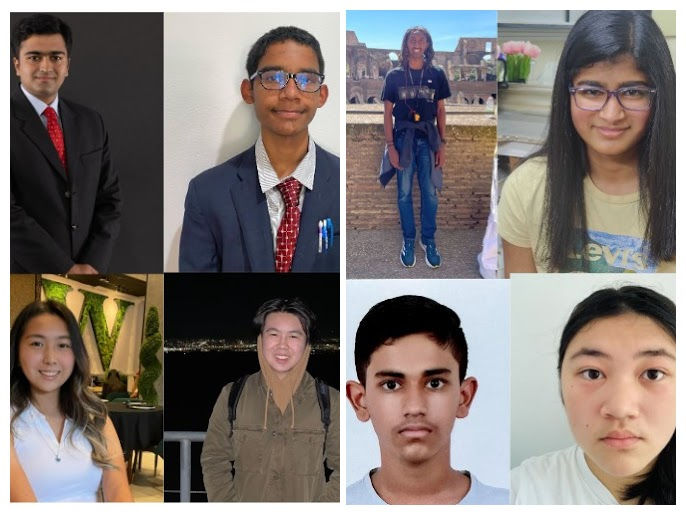
Comments